Managing multifunctional AI requires flexible regulatory approaches, researchers say
New research highlights challenges in regulating AI systems that can perform multiple functions, calling for adaptable oversight methods.
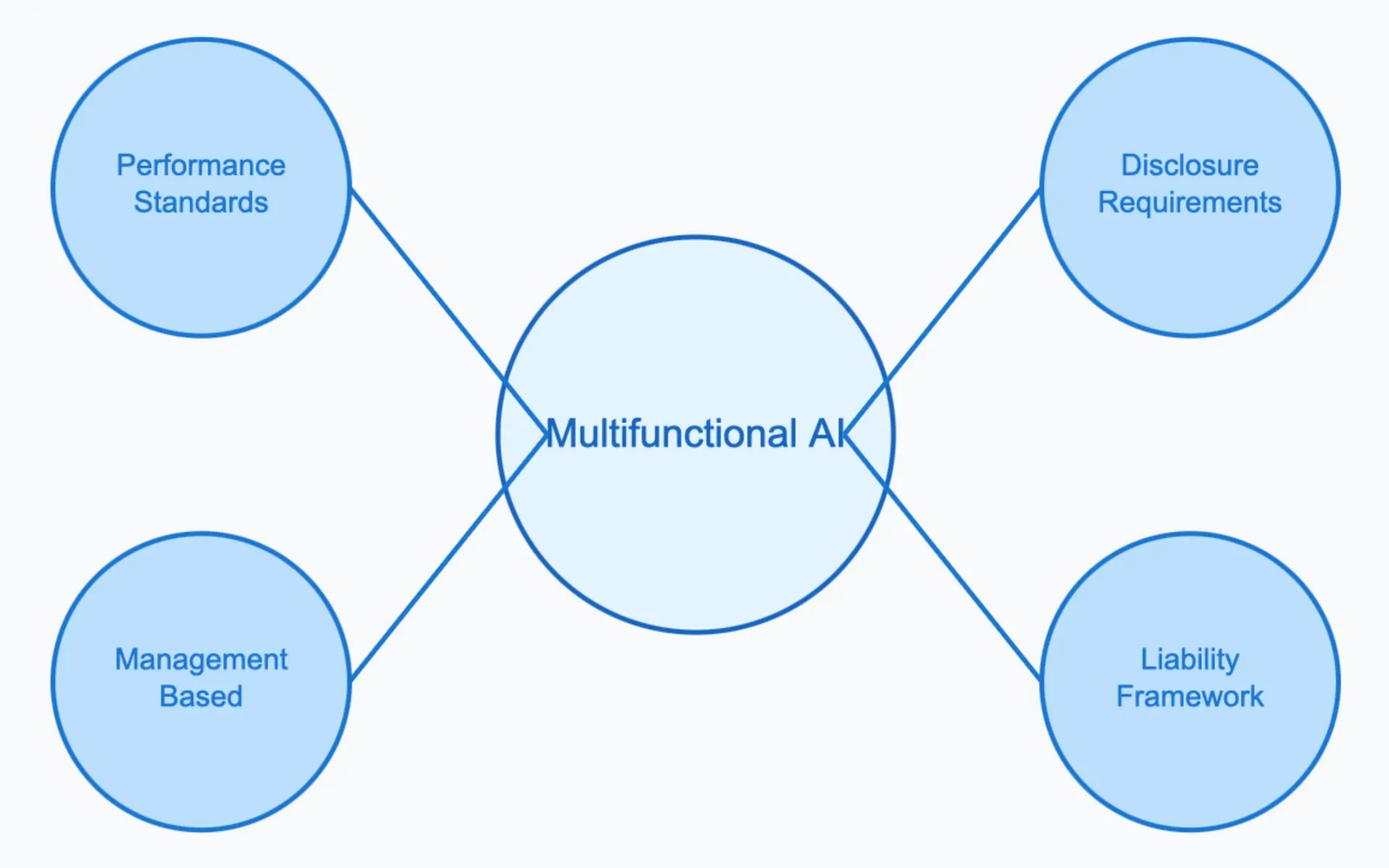
A comprehensive research paper published December 16, 2024, by the University of Pennsylvania Carey Law School examines the intricate challenges of regulating artificial intelligence systems that can perform multiple functions.
According to researchers Cary Coglianese and Colton R. Crum in their paper "Regulating Multifunctionality," released December 16, 2024, foundation models and generative AI tools present unprecedented regulatory challenges due to their vast range of potential uses.
The study compares multifunctional AI to a Swiss army knife - a tool with numerous possible applications that can adapt and change after its initial creation. "Foundation models take multifunctionality to a new level altogether compared with physical tools like the Swiss army knife. Given their extensive range of possible uses, foundation models greatly exacerbate the core regulatory challenge that AI more generally presents: its heterogeneity," Coglianese and Crum write.
The researchers identify three distinct types of heterogeneity that complicate AI regulation: design heterogeneity (different types of AI models), use heterogeneity (varied applications), and problem heterogeneity (diverse risks and challenges).
The paper argues against rigid, prescriptive rules for regulating multifunctional AI. Instead, it advocates for more flexible approaches including performance standards, disclosure requirements, liability frameworks, and management-based regulation.
Management-based regulation emerges as a particularly promising strategy, according to the researchers. This approach requires AI developers to develop internal plans for identifying and monitoring risks, establish protective procedures, and document ongoing risk management efforts.
The study notes specific technical challenges in regulating AI systems. Neural networks, which form the foundation of modern AI, can be configured in numerous ways. Even minor changes in computational hardware or a single neuron can have unpredictable consequences, making prescriptive regulation impractical.
The researchers emphasize the importance of maintaining regulatory vigilance and agility. "Effective AI governance will require constantly adapting, issuing alerts, and prodding action. Regulators need to see themselves as overseers of dynamic AI ecosystems, staying flexible, remaining vigilant, and always seeking ways to improve," the paper states.
Examining current regulatory frameworks, the study points out that existing performance standards and liability systems may prove insufficient for managing multifunctional AI risks. The researchers argue that successful regulation will require substantial resources, including financial support, technological tools, and human expertise.
The paper identifies specific challenges in monitoring AI systems, noting that slight changes in data or training configurations can significantly affect model behavior. These technical intricacies further complicate regulatory oversight.
For public safety considerations, the researchers highlight how foundation models can generate harmful outputs or exhibit biases based on their training data. The paper cites instances where AI models have produced racist content or threatening responses, demonstrating the need for comprehensive oversight.
The study concludes that regulating multifunctional AI demands a multifaceted approach, combining various regulatory strategies while maintaining flexibility to address emerging challenges. This conclusion aligns with recent regulatory developments worldwide as governments grapple with AI oversight.
The research emphasizes that successful AI governance will ultimately depend on building human capital and organizational capacity within both regulatory bodies and private firms. These efforts should facilitate regulatory flexibility while ensuring swift responses to emerging problems.
The paper, published as Research Paper No. 24-55 in the University of Pennsylvania Law School's Public Law Research Paper Series on December 16, 2024, will be included in the forthcoming Oxford Handbook on the Foundations and Regulation of Generative AI.