Building experimentation muscle strengthens marketing effectiveness, says Google
Detailed guide reveals how systematic marketing experimentation creates compound value through improved measurement capabilities.
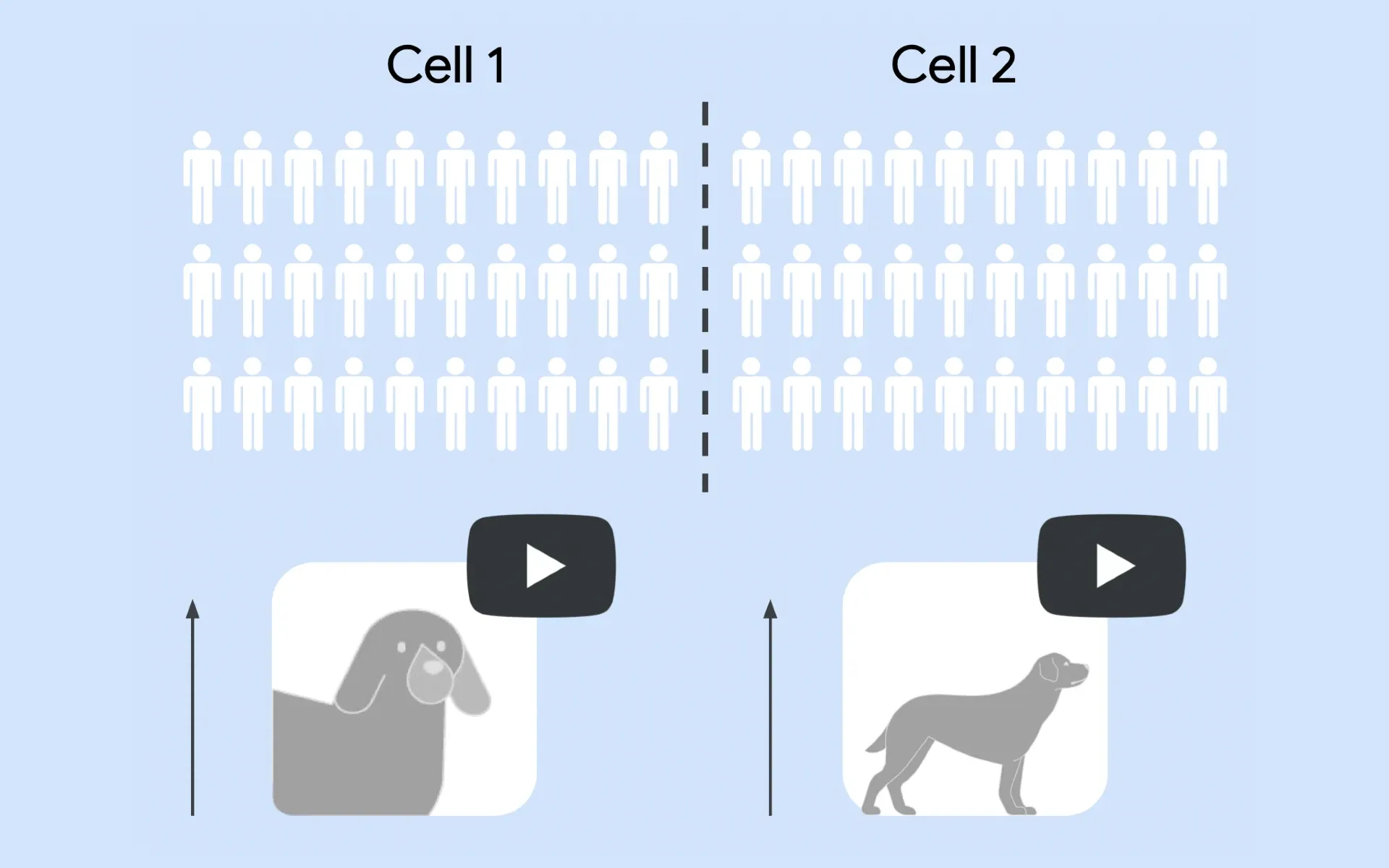
Marketing experimentation can serve as a competitive advantage by enabling organizations to grow faster than their peers, according to a comprehensive guide released by Google. The document outlines how companies can build what Google terms a "measurement muscle" through systematic testing of marketing activities.
The value of this experimental approach manifests in concrete numbers. According to Google's analysis, organizations conducting monthly experiments with 5% effectiveness improvements achieve a 3.1x increase in returns over a two-year period. Companies running quarterly experiments see a more modest but still significant 1.4x improvement in the same timeframe.
"Marketing and advertising, if executed well, can serve as a competitive advantage for any company," states the guide. "But how can we determine whether marketing is being executed well? That's where experiments come in."
The document emphasizes that experimentation enables organizations to move beyond correlation to establish causality in marketing effectiveness. This capability proves crucial for answering fundamental questions about media performance, including optimal budget allocation, audience targeting, and creative strategy.
To build this experimental capability effectively, Google recommends assembling what it calls an "experimentation squad." This team requires four distinct skill sets: a creative strategist to generate testing ideas, a business analyst to prioritize experiments based on potential impact, a data scientist to ensure statistical validity, and a decision-maker to allocate resources and implement findings.
The methodology emphasizes four critical characteristics of high-quality experiments. First, experiments must link questions to specific actions. The guide warns against conducting tests purely for knowledge gathering, stating that every experiment should connect to concrete business decisions.
Second, experiments need to focus on meaningful metrics that tie directly to business objectives. Google introduces an effectiveness-efficiency framework that balances growth indicators against cost constraints. This approach helps organizations maximize returns while maintaining profitability targets.
Statistical considerations form the third pillar of the methodology. The guide emphasizes the importance of proper experimental design, including power analysis to determine adequate test duration and sample sizes. This mathematical rigor helps organizations avoid drawing incorrect conclusions from insufficient data.
Finally, experiments must account for broader context. The guide cautions against over-generalizing results, noting that marketing effectiveness varies across time periods, geographical regions, and economic conditions. This understanding drives the recommendation for regular testing cycles rather than relying on historical data.
For organizations beginning their experimental journey, the guide recommends starting with clear business questions linked to organizational key performance indicators. This approach ensures that experimental findings translate directly into actionable insights that drive business growth.
The document also introduces the concept of metric waterfalls, which help organizations handle data sparsity challenges. This framework enables companies to make informed decisions even when primary metrics lack sufficient data by incorporating correlated secondary and tertiary indicators.
The methodology emphasizes that building experimental capability requires sustained commitment. According to Google, the compound effect of regular testing creates a "competitive advantage that allows them to grow more quickly than their peers."
This systematic approach to experimentation represents a shift from traditional marketing measurement. Rather than relying on correlation-based analyses or periodic effectiveness studies, organizations can build continuous learning capabilities that drive ongoing optimization of marketing investments.
The guide concludes by highlighting the dynamic nature of marketing effectiveness, emphasizing that experimental results remain valid only within specific contexts. This reality underscores the importance of building robust experimental capabilities that enable organizations to adapt rapidly to changing market conditions.
Through this methodical approach to experimentation, organizations can develop what Google describes as a "measurement muscle" that provides sustainable competitive advantage in increasingly complex marketing environments.
Understanding the KPI waterfall: A data-driven approach to marketing measurement
The challenge of measuring marketing effectiveness often lies in data scarcity, a problem Google addresses through what it calls the "KPI waterfall" framework, according to its experiments playbook. This structured approach helps organizations balance the competing demands of statistical significance and business relevance.
At the heart of this methodology lies a fundamental challenge in marketing measurement. As stated in the guide, primary business metrics, such as revenue or customer acquisitions, frequently occur too infrequently to provide statistically significant results within reasonable testing timeframes.
Google illustrates this concept through a case study of an automotive company. According to the document, car purchases represent the ultimate business outcome but occur too rarely to serve as reliable experimental metrics. The company needed to identify alternative measurements that could indicate effectiveness while maintaining sufficient data volume for statistical validity.
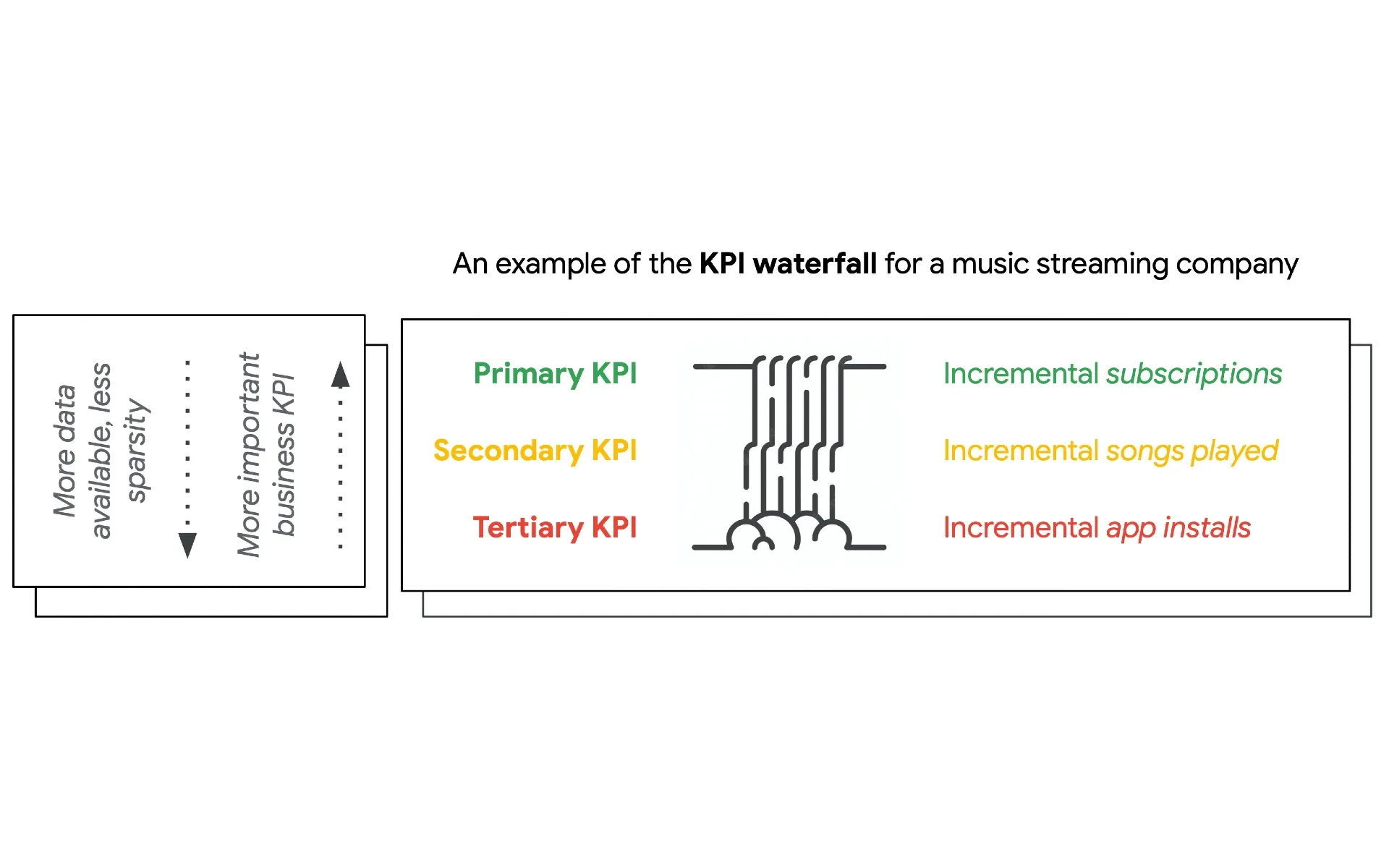
KPI waterfall framework
The KPI waterfall framework addresses this challenge through a three-tiered metric system. According to the guide, each tier serves a specific purpose while maintaining clear connections to business objectives. A music streaming service example demonstrates this hierarchy: subscriptions serve as the primary KPI, songs played as the secondary metric, and app installs as the tertiary indicator.
The methodology emphasizes the relationship between metric frequency and business importance. Higher-tier metrics typically carry greater business significance but occur less frequently. Lower-tier metrics generate more data points but may have less direct business impact. The framework helps organizations navigate this trade-off systematically.
Google provides a detailed example through ChewyTreats, an online dog food retailer testing different video creative approaches. The experiment tracked three metrics: incremental conversions, add-to-cart actions, and website visits. When primary and secondary metrics showed directionally positive but statistically insignificant results, the more frequent tertiary metric of website visits provided actionable insights.
The framework's practical application becomes evident in the results analysis. According to the document, ChewyTreats' incremental conversions showed ranges of [12k-16k] versus [13k-17k] between test groups, while website visits demonstrated clear statistical separation at [100k-130k] versus [140k-165k]. This data volume enabled confident decision-making despite primary metric uncertainty.
Critical to the framework's success is the correlation between metric tiers. The guide emphasizes that lower-tier metrics must demonstrate strong relationships with primary business outcomes to serve as valid proxies. Organizations need to validate these correlations before incorporating metrics into their measurement framework.
The methodology also addresses the temporal aspect of marketing measurement. According to the guide, higher-funnel metrics often provide earlier indicators of campaign performance, enabling faster optimization cycles while maintaining alignment with ultimate business objectives.
Google's framework includes specific guidance for metric selection. The document recommends choosing secondary and tertiary metrics that balance three key criteria: data availability, business relevance, and proven correlation with primary outcomes. This structured approach helps organizations avoid the pitfall of optimizing for easily measured but commercially irrelevant metrics.
The KPI waterfall framework represents a significant advancement in marketing measurement methodology. By systematically incorporating metrics of varying frequency and business impact, organizations can maintain both statistical validity and commercial relevance in their experimental programs.
Through this structured approach to metric selection and analysis, companies can build more robust measurement capabilities while staying focused on ultimate business outcomes. The framework provides a practical solution to the persistent challenge of data sparsity in marketing experimentation.